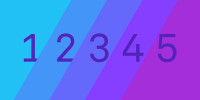
An enterprise checklist for synthetic data success

We’re now almost five years into GDPR, and the ICO is continuing to ramp up its enforcement efforts. Both the frequency and severity of fines have increased, and of the entire sum of fines, 90% comes from infractions within the last two years.
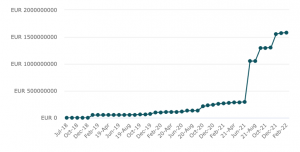
Whilst this backdrop can look bleak - the landscape is primed to power new innovation, new ways to deliver on those bigger targets, and new ways to make your data available and usable.
Enterprises across regulated industries are already making strides in adopting new technologies to help them improve access and speed of procuring data. One of the technologies is synthetic data. But as with any nascent, enterprise technology, it requires a step by step implementation.
Our team has put their heads together to offer our five best tips to help you ensure your synthetic data project is a success.
1. Clean, analyse, clean (your data, that is)
Firstly, ensure you can access the data. One of the biggest timesinks to any data project, synthetic data or otherwise, is customers not being able to share their data with providers.
Next, do your data analysis. Understand your data: the schema, what it’s made up of, and what you can and can’t do with it. Factor in data regulation (GDPR, CCPA) as well as data cleanliness - ensuring data is consistent and free from errors.
If needed, clean your data. The main reason projects are usually held up is due to dirty data. That said, ensure you keep the spread of data points so as not to add bias to your dataset.
It’s not always the most interesting part of the project but without sorting these crucial first steps, your plans will be derailed: garbage in, garbage out (GIGO).
2. Define a direction. What are you actually trying to achieve with synthetic data?
Know where you want to go with your synthetic data strategy - and who you need to make it a success.
Focusing on specific use cases is an achievable way to incrementally deploy new technology throughout your organisation but not knowing your desired outcome will mean your project will lose momentum and could be de-prioritised.
Know the hypothesis you are trying to prove and share it with senior stakeholders.
Ensure to return to the original goal at the end of the project and assess performance to prove value and support with buy-in for future work.
Take Nationwide Building Society for example: the team established that the primary value of synthetic data was to reduce the risk of live data outside of the live production environment to reduce risk of a data breach. They also collated a list of secondary value items.
The NBS team created a value proposition canvas for synthetic data, mapping out key roles, gains, pain reducers, expectations and customer jobs that needed to be factored in, as well as carrying out analysis of the alternatives. This document serves as the north star for their project.
3. Choose an easy yet impactful use case to start
From moving to the cloud, to training AI models tackling customer churn eating away at your quarterly targets, there are lots of use cases that synthetic data can provide a viable solution to.
After setting your ideal outcome, choose a use case that easily demonstrates ROI. Usually this is based on time saved, or incremental revenue generated.
Training AI and ML models is usually a good use case to kick off with as the data will likely be in reasonable condition, making step 1 quicker to execute. Vodafone Group started with a use case that used synthetic data to train and test machine learning models. The complete training phase of the data generator took 1 minute, compared to waiting several weeks to get access to production data or create a test dataset manually.
4. Define metrics before starting
It goes without saying that any new initiative warranting budget and resource must be tied to a business outcome, and project owners should be prepared with the metrics to show its performance.
And, beyond just thinking about and mapping what metrics you are setting, consider how you will both display them and report on them.
Visually communicating and demonstrating to your team the efficacy and reward of new projects is paramount to not only validate your initiative, but to also set yourself up for a more streamlined approval process in the future.
When it comes to synthetic data roll outs, we commonly see customers needing to show proximity of synthetic data vs original data, utility, and time taken to synthesise. We help our customers define the best metrics, and support them in building reports using the Hazy Hub and report templates.
5. Select a provider that bakes privacy into its technology
Whilst metrics are crucial to provide business impact and data utility, metrics are not enough to ensure that the data created is anonymous.
One of synthetic data’s strengths is that the connection to the original datapoint is severed, meaning it retains its referential integrity whilst protecting the PII (and falling outside of some data regulation such as GDPR).
However, this is not a standard aspect of all synthetic data generation; it needs to be built into the technology. Specifically, the generative models need to be trained with built-in Differential Privacy (DP), (which provides mathematically provable privacy guarantees).
Select a provider whose technology has DP built in to ensure your customer data remains safe and usable.
If you’d like to make your data available and fast-track your 2023 data strategy, get in touch.