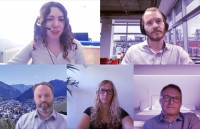
techUK panel: Five things you may not know about synthetic data

We spoke at a recent techUK panel alongside a number of other industry experts about the latest thinking on synthetic data.
Below, we’ve summarised five areas of thinking from the conversation that may help you continue your understanding of where and why synthetic data is so valuable and how it applies to enterprise businesses.
With our thanks to the other participants and techUK for facilitating:
- Dr. Martin O'reilly, Director of Research Engineering, The Alan Turing Institute
- Jeremy Poulter, Business Development & Solutions Director, Defence and National Security, Microsoft UK
- Alexandra Ebert, Chief Trust Officer, Mostly AI
- Laura Foster, Head of Tech & Innovation, techUK
The most valuable data is becoming the hardest to access
Medical research is patently one of the most valuable activities any society can pursue. However, the industry must do everything in its power to achieve breakthroughs while respecting regulation, preserving privacy and ensuring patient data is kept secure.
As a result, access to data is getting harder and harder, limiting the effectiveness of even the most powerful learning models.
Without using synthetic data to surmount these issues, it’s hard to see how we will get the most from the enormous potential of these systems.
You can create 100% synthetic models from nothing - if you approach it right
For better or worse, there is no godlike algorithm that can synthesise perfect synthetic data by itself. It relies entirely on the data owner or data consumer setting context or boundaries that are laser-focused on the requirements of your use case.
However, the good news is: if you are able to focus truly on what it is you will need from the final data to achieve your goals, you can use generative models to produce it while retaining its representation of the real world.
There are several key ideas to getting this right, but a good example is “narrative fidelity” - the degree to which a story fits into the observer's experience. Within synthetic data, this means preserving the context between data. It’s not enough to capture static correlations and complicated statistics, so firms like the Alan Turing Institute are researching models that inject some ‘common sense’ into the structure of the model.
Financial services is leading the way
In many areas of tech innovation, the rigorous limitations faced by the financial services industry can leave it to lag behind businesses that don’t face such responsibility. Added to financial regulation, GDPR also inhibits many financial services using and internally sharing the data they have.
The significant increase in fines for GDPR non-compliance, from €158.5 million in 2020 to just under €1.1 billion in 2021, is a signpost for what’s coming ahead if organisations continue to ignore GDPR laws.
However, because synthetic data is such a fundamental way to overcome many of those challenges with data, even the biggest financial services teams in the world are leading the way with use of this technology. This is a good demonstration of what we might expect to see in many other industries as the use of synthetic data continues to grow.
You must still be aware of bias
As long as we live in a world of human beings, we will have to tackle unconscious bias around data. And because of the authenticity of good synthetic data, naturally this challenge remains. This is a particularly complicated area because it overlaps with the concept of “fairness” - a confusingly broad and slippery term in machine learning.
At Hazy, we advocate for “differential privacy”, because it’s the only provable way your generative models can defend against attacks, without sacrificing a useful level of quality. Everyone working with synthetic data must pay close attention to this area, to make sure they can look their customers in the eye and assure them they are doing the right thing to treat their data properly.
Consistent with ICO guidance, our approach takes into account the concept of identifiability in its broadest sense. It does not simply focus on removing obvious information (e.g., direct identifiers), instead, it sufficiently anonymises the data to reduce the possibility of:
- any individual being distinguished from other individuals (singling out or individuation).
- one or more other datasets or records (whether or not publicly available) being combined with the synthetic data and thereby enabling the identification of an individual (linkability).
- a reasonably competent intruder employing investigative techniques and having access to appropriate resources (e.g., the internet, libraries, public documents) being able to achieve identification if they were motivated to attempt it (motivated intruder test).
Synthetic data unlocks the future for machine-learning
This is an incredibly exciting time. Many concepts that seem like science fiction require such volumes of data that the idea of getting enough while still respecting privacy and security is almost imaginable.
The only way society is going to be able to move into those areas at scale is synthetic data. That’s the path to being able to create truly expansive, detailed virtual worlds, where parameters can be changed in real time as the use case requires.
Check out the full talk below
We had a great time on this panel, and highly recommend you tune into the full talk here.
This webinar was a part of techUK’s future vision series.