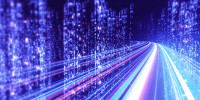
Synthetic data unlocks true cross-organizational data portability

There’s no doubt organizations are collecting more data than ever. In fact, 90 percent of all data has been created in the last two years. Yet, with more data, more problems. Organizations of all sizes are consistently struggling to access and understand all that data.
The only way to unlock the value of all that data is to enable it to become portable — across silos, boundaries and borders. But it can’t come at a risk of leaking private information. Organizations are only beginning to realize the privacy implications of how massive amounts of personal data is pieced together to create patterns that give valuable insights into user behavior, but run the risk of data exposure.
Organizations must balance the need for data agility and innovation with governance, compliance and security.
Over the last few years, artificial intelligence-trained synthetic data has arisen as a high-quality drop-in for real data. Synthetic data retains the statistically representative behavioral patterns of the raw data it’s trained on, without running the same risk of re-identification as traditional data masking and anonymization methods. It gets the necessary value out of the real data — usability, compliance, and access to innovation — without using any real data. And synthetic data achieves all this with a measurable level of privacy, something that appeases enterprise information security teams.
Synthetic data enables organizations to share the insights of data across different departments, divisions and with third parties, without risk of data leakage. Synthetic data allows for data portability and breaks down geographical data silos for truly cross-organizational analytics. It allows organizations to evaluate potential vendors, services and algorithms in innovation sandpits. And synthetic data allows orgs to increase speed to decision making, without risking or getting blocked on real data.
This is what Logic 20/20’s Adam Cornille, synthetic data generator Hazy’s Harry Keen, and Microsoft’s Tom Davis dove into during last month’s webinar on Smart Synthetic Data. We encourage you to listen to this dynamic conversation around privacy and the potential of data portability. And then in this piece we introduce you to the potential of synthetic data to drive it.
The burden of cross-enterprise data
We no longer live in a world where organizations build everything from scratch. There’s not enough time for enterprises to design, build, and test features at a rate that can compete with disruptors like in fintech. It’s become best practice to partner with third-party vendors to leverage their tools and services. This can be anything from access to the cloud to SaaS tools like customer relationship managers (CRMs) to strategic partnerships.
However, heavily regulated multinational institutions like banks are struggling not only to compete with these up-and-coming challengers, but they are also dealing with cross-border and cross-organizational laws and privacy regulations.
Nationwide Building Society was facing this struggle, when looking to evaluate potential innovation partners. When they applied data masking techniques, they discovered that, when paired with not-related sources, like open data, it was possible to de-anonymize that data. That was not an acceptable risk.
Data masking and data anonymization are widespread practices that are notorious for running this risk of re-identification. These processes also fail to meet most quality standards, as anonymization fails to preserve the key statistical relationships and patterns in the original data.
In the end, Nationwide settled on synthetic data generated by Hazy as this was shown to preserve the key behavioral signatures and relationships between data points, while exposing none of the original data.
At Nationwide, Hazy synthetic data had to stand up against four criteria:
- Evaluation of potential third-party partners — Nationwide partners with third-party integrators to create improved services for their customers. To achieve this, Nationwide needs to share realistic data to truly evaluate the technical offering of these external apps and tools.
- Risk mitigation for the data engineer — When a developer creates a batch feed or intake, up until a few months ago, that was done on live data. Developer environments aren’t as secure as production environments and risk leaking data.. Using synthetic data mitigates this risk.
- Reusability for data analytics teams — Data anonymization often needs to be repeated manually for every business application and is therefore resource-intensive. Nationwide was looking for a synthetic data tool that could generate data quickly and that could be used flexibly across multiple business applications.
- Safe sequential data for behavior analytics — Find a way to analyze behavior patterns over time without risking personal information.
Most importantly, this had to be done in an auditable process, ensuring the statistical quality of the data was measured and maintained.
In the end, Hazy synthetic data generation was chosen for how it was proven to limit Nationwide’s risk of data exposure and data regulation fines, while still retaining measurable quality using Hazy’s advanced quality assessment metrics.
It used to take the Nationwide strategic partnership team six months to evaluate new data technology, services and vendors. With Hazy, now it just takes them three days.
The world-changing potential of time-series synthetic data to drive data portability
The Holy Grail of synthetic data has always been sequential data. This can be strings of transactions, medical records, stock market movements, weather patterns, pandemic spread, or anything else where the order and time of events matters.
We aren’t talking about just one row. Banks have thousands of time-bound data attributes sometimes over decades of customer history. This means they are sitting on terabytes of data, that is often locked within secure silos, unavailable even for in-house data scientists to build value on top of.
Obviously this amount of data runs an even greater risk of leaking deeply personal information. Generating safe synthetic data that preserves both timelines and privacy has dramatic potential to enable cross-organizational and cross-industry collaboration to solve some of the biggest problems at a world scale.
Hazy recently unlocked how to generate sequential and time-series synthetic data. This is a monumental breakthrough with a plethora of use cases, some already in effect. One customer is using artificial transactional data to evaluate innovation partners in building a behavioral, research-driven digital banking solution that helps individual consumers make better financial decisions. Nationwide’s rapid innovation team is similarly using Hazy’s synthetic transactional data to give to third parties tasked with analyzing behaviors of users in order to pinpoint more financially vulnerable customers.
Synthetic data also opens the potential for data pools and the ability to collaborate with other organizations and nonprofits to examine together seemingly insurmountable obstacles like patterns of fraud detection and evaluating alternative data to open greater access to banking.
Synthetic time-series data could be applied to allow more open, but secure sharing of information, which can lead to more accurate cancer detection, insurers offering better preventative care, and faster identification of money-laundering — without risking privacy leaks.
Sequential synthetic data can even be applied to prepare for the next pandemic or other unforeseen events.
Artificial intelligence has only begun to interpret this overabundance of data in order to try to tackle these immense challenges. Until now data regulations have limited access to data sharing and central data repositories. Synthetic data pools are the best and perhaps only way to compliantly bring together industry leaders to collaborate to solve some of the world’s biggest problems.
We know that cross-organizational and cross-sector data portability is the only way to truly unlock the value of big data. Now we know synthetic data is the only way to make that happen.
This post first published on our partner Logic 20/20’s Business Insights Blog.