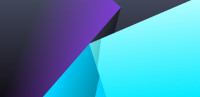
Anonymisation vs Pseudonymisation vs Synthetic Data

Anonymisation or pseudonymisation?
Anonymisation is a set of processes and methods that can be applied to a dataset in order to ensure that the data it contains cannot be connected to an individual or entity from which the data comes.
Psuedoanonymised information uses a technique that can improve user privacy by replacing or removing the most identifying fields in a data set. This may involve replacing names or other direct identifiers which can be easily attributed to individuals with, for example, a reference number. Pseudonymised data can reduce the risks of identification of data subjects and help companies meet some data protection obligations.
In essence, this is just a security measure and does not change the status of the data as personal data. Sharing any data that has undergone pseudonymisation still has to be done strictly in accordance with GDPR standards. For instance, if the data subject requests access and asks to be removed from the data, the appropriate procedures have to be followed and response should be fulfilled without undue delays, regardless of any attempt to pseudonymise any personally identifiable information
However, GDPR does not apply to personal data that has been anonymised if performed in a correct and robust way. Anonymising personal data can therefore provide a more effective method for businesses seeking to limit the risks when sharing data, whilst protecting identifiable data too.
What else can I do to enhance data privacy?
There are a number of privacy enhancing technologies on the market, some of them are expensive and require enterprise grade technical capacity, but others are fairly simple and affordable for SMEs and even young startups.
Selecting the right combination may be a challenge, despite the fact that the knowledge of different methods is quite evolved and there's a lot of available information.
Techniques include:
-
Data Masking - a method of creating structurally similar but inauthentic versions of an organization's data that can be used for purposes such as software testing and user training. The purpose is to protect the actual data while having a functional substitute for occasions when the real data is not required, such as generating dummy data for software development.
-
Differential Privacy - allows companies to learn more about their users by maximizing the accuracy of search queries while minimizing the chances of identifying personal records. Differential privacy requires filtering data, adaptive sampling, adding noise by fuzzing certain features, analysing or blocking intrusive queries.
-
Homomorphic Encryption - a method for performing calculations on encrypted information without decrypting it first. Why should we care about arcane computer math? Because it could make cloud computing a lot more secure. It's not quite ready for your email though—right now it makes processes literally a million times slower if you use it. Plus, its use significantly limits the amount of operation or processing that can be done on the data whilst keeping the real data intact.
-
Synthetic data - this is Hazy's core capability: generating artificial data that contains no real data or customer information whilst preserving all the insight, patterns and correlations in the source data.
While there are often weaknesses in anonymised data, synthetic data — especially backed with differential privacy — offers a higher level of utility without the risks of re-identification.
Use cases for synthetic data
Smart synthetic data generation has rapidly advanced over the last few years alongside advances in the machine learning algorithms that are driving it. Smart synthetic data is data that is completely artificial but maps to the patterns of behaviour of the original data, while fully protecting privacy. As you can imagine, there are many use cases for synthetic data that enable businesses to get the value out of data without any of the risks.
Working with synthetic data, as opposed to real data, eliminates the vast majority of attack vectors, because synthetic data is fake data and so there's no real data or customer information to leak. In addition, synthetic data can be combined with other privacy techniques for additional guarantees.
For example, synthetic data generator models can be trained to be differentially private, and data can be generalised either before or after training a generator.
The challenge is making the synthetic data smart enough to carry through the insight and information from the source data. This starts with matching simple patterns like distributions of values in a column, through to complex modelling of implicit relations and sequential data.