Data
Hazy generates sample based synthetic data, based on a statistical analysis of a source dataset. This source data must be structured data in tabular / relational form. This can be ingested from files or databases.
Hazy has support for multi-column and multi-table distributions, sequential data and complex business logic. See the full list of data features.
Sampling¶
Hazy generates sample based synthetic data. This means that Hazy’s synthetic data is not just based on a schema or generated out of thin air. It is based on the statistical analysis of a source data set.
Ingesting this source data is the start of the Hazy process. The source data is ingested, analysed and then a Generator is trained to learn all of its patterns and correlations. This Generator is a serialisable object, containing compressed representations of the patterns and correlations in the source data.
- Data ingestion
- Data processing / cleaning
- Generator training
The process starts by ingesting the source data at the start of the Hazy process. Data processing deals with all necessary transformations required before feeding the data to train Hazy Generators. These can be normalization, binarization, 1-hot encoding, logarithm scaling and so on. The Generator is trained to learn all the complex relations between different columns in the data (distributions, correlations and so on). This Generator is a serialisable object, containing compressed representations of dependencies between the variables. For PrivBayes, the Generator consists of a Bayesian Network, for Synthpop the Generator is a collection of decision trees.
Synthetic data is then generated by sampling from the Generator and propagating the signal through the network, for example, generating values for a column by sampling from its distribution estimate:
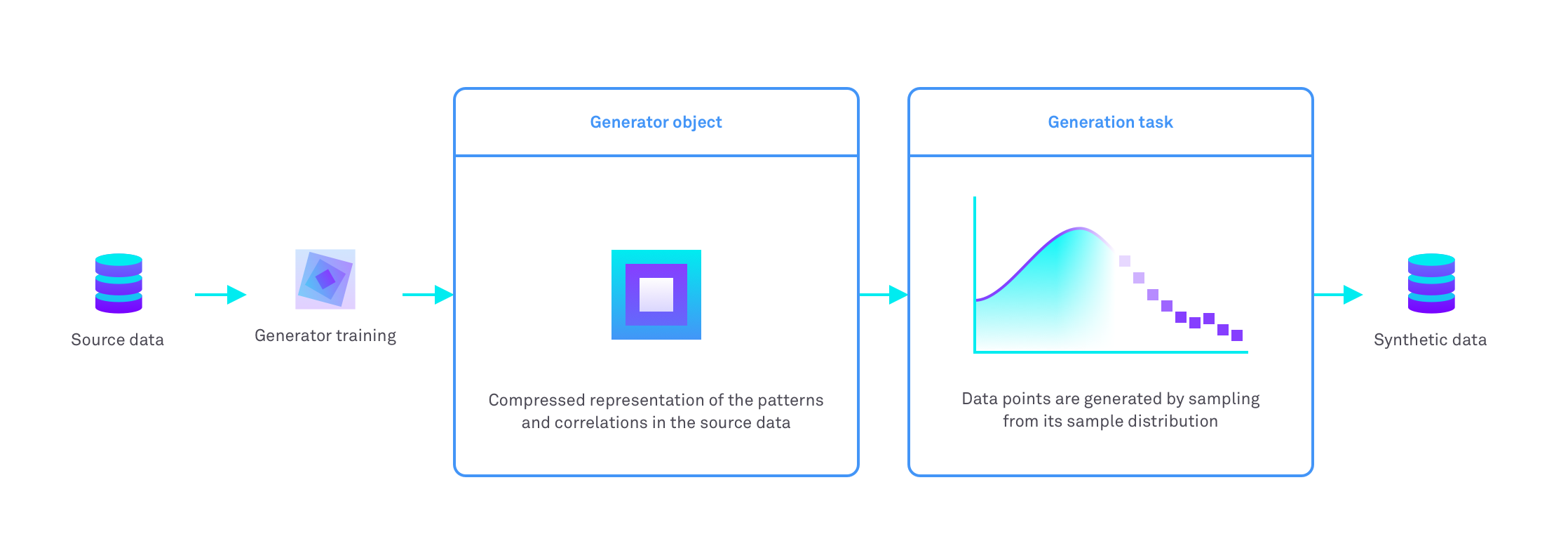
The synthetic data is guaranteed to preserve the statistical properties of the source data whilst still being safe. A concept that is detailed in the differential privacy section.
Features¶
50+ Data types¶
Hazy has a wide range of supported types. For dealing with free/unstructured text see here.
Multi-column distributions¶
Hazy models not only the distributions of single columns, but also the relationships between columns.
Multiple tables with referential integrity¶
Hazy models can cope with data extracted from multiple tables that require some level of referential integrity to be preserved. A number of different table types are supported.
Reference tables¶
These are tables within a database schema that contain information the user does not want to be synthesised, these will be recreated exactly as they were supplied during training. This presents a privacy risk so should only be used with tables that the user does not care about applying privacy to. See reference tables for more information regarding usage.
Automatic data type detection¶
Our analysis step detects a wide range of data types, constraints and formats. It also calculates a set of statistics that can aid configuration.
Business logic¶
Hazy supports a wide range of business logic. Continuous data columns can be configured to be calculated by user defined formulas. They can also have min/max bounds set - either by a constant or by other columns. See here for which Hazy data types these can be applied to.
Hazy ID formats can also be used to encapsulate complex business logic.
A large set of data types support being assigned to entities such as people
, locations
and categories
. This allows coherent data to always be produced ie. typically male first names will be statistically assigned male gender. Post/zipcodes will be generated within the correct regions. Enums and sub enums can be made to not violate business logic even when noise is introduced into the process.
Sequential tables¶
Sequential data, also called time series or transactional data, is structured data with correlations between rows as well as columns, for instance, bank transactions. Sequential data is challenging to synthesise, as the relationships between rows are often implicit and can have long causation chains (for instance, spending patterns over the Christmas period can be related to spending patterns that occurred in Summer). The trend’s underlying distributions in the data can also be dynamic, which makes them hard to predict and generate. For example, when a life event occurs, like marriage or a change in employment status, the patterns of bank transactions may change considerably.
Hazy has a number of different approaches to modelling and generating sequential data. These include, bootstrapping, windowing, temporal Generative Adversarial Networks (GANs), Auto-Encoders and sequential Synthpop.
See sequential tables to understand how to configure these in the Hazy Hub.
Database subsetting¶
Database subsetting is a way of sampling a database. You train on a smaller amount of data, which offers a faster feedback loop and saves time overall. It also reduces the cost of hardware or cloud compute needed to carry out training. You can find out more here.
Limitations¶
Imbalanced data / outlier detection¶
In order to make privacy guarantees, Hazy applies "noise" to the data, in the form of ε
differential privacy level and can "cut out" outlier data points when applying disclosure risk thresholds. As a result, imbalanced data sets with low frequency signals and downstream modelling and analysis tasks that aim to detect outliers can suffer from a loss of utility.
Hazy has bespoke support for conditional generation, using our deep learning algorithms to apply statistical control and rebalancing of the output synthetic data set. This can help to address limitations with maintaining quality for imbalanced data, for instance, in fraud detection and cyber-security.
If you need help with any of the above, please contact us.